GWAS-MAP: Biomarker and intervention target discovery platform
Note: we now provide access to the GWAS-MAP platform for free to anyone researching the novel coronavirus (SARS-CoV-2 or COVID-19). If you are interested, please feel free to contact us via e-mail (info@polyknomics.com).
Over the last decade, thousands of complex traits, including common diseases, have been analyzed using genome-wide association studies. At the same time, progress of high-throughput molecular technologies allowed for the study of genetic determinants of different “omics”, such as metabolomics, transcriptomics, glycomics, and proteomics.
Our platform, GWAS-MAP (Figure 1), integrates results of genome-wide association and functional genomic analyses of millions of samples. For many years, we have collected, inspected the quality, and harmonized data that was spread across numerous scientific publications.
The platform uses the genome as a causality “anchor” and common reference which allows linking of results obtained for disease(s) of interest, their endophenotypes, and a wide range of molecular measurements. Having these big data, we apply novel analytical methods to develop etiologic hypotheses, discover candidate risk assessment biomarker, and biomarkers that may help stratifying the patients into different molecular subtypes of disease. The results may pave the route to better prognosis, prediction, and diagnosis.
Our platform can also suggest targets for therapeutic intervention, including candidate drug targets, and candidate modifiable factors that may be modulated by known drugs or lifestyle interventions. The platform can be used to support the process of for drug re-purposing, and as a part of the process of target discovery and pre-clinical phases, contributing to target validation, insight into efficacy, safety, and a range of potential side-effects.
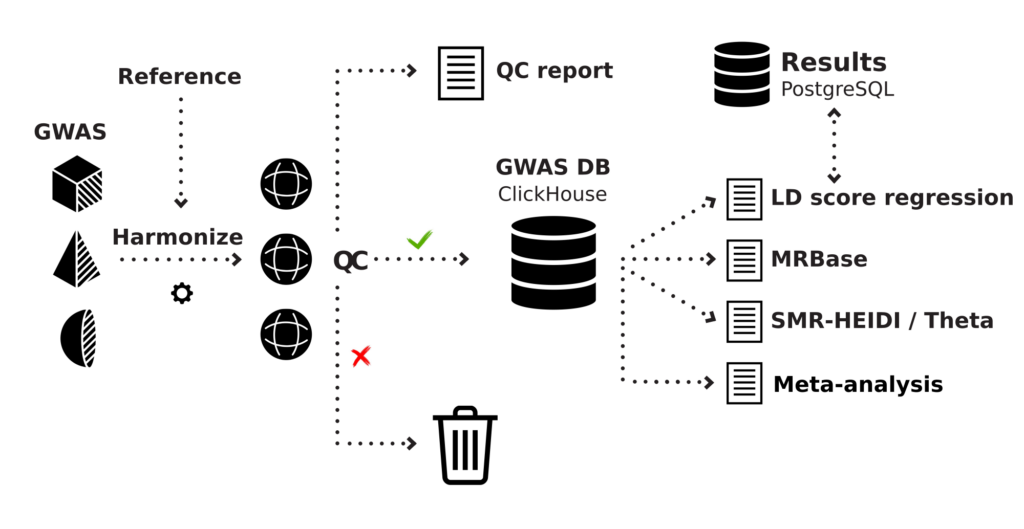
Figure 1: Schematic overview of the GWAS-MAP platform architecture.
At the moment, the high-performance database that is distributed as a part of GWAS-MAP, stores data on genome-wide studies of hundreds of human diseases, thousands of complex and quantitative traits and thousands of “omics” (metabolomics, proteomics) phenotypes. It also contains data from more than a million region-wide association studies (mostly, cis-eQTL from transcriptomics studies). In total, the database stores more than 70 billion trait-variant associations. This number is expected to grow to at least 100 billion associations by the end of 2020. Many of the data sets we have consolidated in GWAS-MAP are not available in a usable format elsewhere.
The platform implements popular methods for joint and meta-analysis of results of genome-wide association studies. These include methods for meta-analysis1, methods that allow estimation of genomic correlations2,3, analysis of association signal colocalization4,5, methods to infer causal relations between traits6, and methods for building predictive models7,8. On clients’ request, we can implement other methods in the system as well.
The GWAS-MAP and its elements were used as a backend for storage or results, access and visualization, as well as post-GWAS analyses in research, published in leading genetic and medical journals. With the help of the platform, scientists has studied back pain9–11, varicose veins12, healthspan13, protein glycosylation14, and the transcriptome of multiple cell types5.
The database management systems of GWAS-MAP is used in online platforms such as GWASArchive and PheLiGe, that were developed by PolyKnomics BV.
To manage big genomic data effectively, the GWAS-MAP platform employs the ClickHouse, a high-performance distributed database management system for data analytics. For best performance, GWAS-MAP needs to be deployed using a specifically optimized architecture that consists of at least three types of nodes integrated with a high-speed network infrastructure. The GWAS-MAP platform can be deployed on the hardware of PolyKnomics BV (platform as a service model; our servers are located in Amsterdam, The Netherlands), in a cloud, or on the client’s hardware.
-
- de Bakker, P. I. W. et al. Practical aspects of imputation-driven meta-analysis of genome-wide association studies. Hum. Mol. Genet. (2008). DOI:10.1093/hmg/ddn288
- Bulik-Sullivan, B. K. et al. LD Score regression distinguishes confounding from polygenicity in genome-wide association studies. Nat. Genet. 47, 291–295 (2015). DOI:10.1038/ng.3211
- Bulik-Sullivan, B. et al. An atlas of genetic correlations across human diseases and traits. Nat. Genet. 47, 1236–1241 (2015). DOI:10.1038/ng.3406
- Zhu, Z. et al. Integration of summary data from GWAS and eQTL studies predicts complex trait gene targets. Nat. Genet. 48, 481–7 (2016). DOI:10.1038/ng.3538
- Momozawa, Y. et al. IBD risk loci are enriched in multigenic regulatory modules encompassing putative causative genes. Nat. Commun. 9, 2427 (2018). DOI:10.1038/s41467-018-04365-8
- Hemani, G. et al. The MR-Base platform supports systematic causal inference across the human phenome. eLife 7, (2018). DOI:10.7554/eLife.34408
- Vilhjálmsson, B. J. et al. Modeling Linkage Disequilibrium Increases Accuracy of Polygenic Risk Scores. Am. J. Hum. Genet. (2015).
DOI:10.1016/j.ajhg.2015.09.001 - Lloyd-Jones, L. R. et al. Improved polygenic prediction by Bayesian multiple regression on summary statistics. Nat. Commun. (2019). DOI:10.1038/s41467-019-12653-0
- Suri, P. et al. Genome-wide meta-analysis of 158,000 individuals of European ancestry identifies three loci associated with chronic back pain. PLOS Genet. 14, e1007601 (2018). DOI:10.1371/journal.pgen.1007601
- Freidin, M. B. et al. Insight into the genetic architecture of back pain and its risk factors from a study of 509,000 individuals. Pain 160, 1361–1373 (2019). DOI:10.1097/j.pain.0000000000001514
- Elgaeva, E. E. et al. ISSLS Prize in Clinical Science 2020. Examining causal effects of body mass index on back pain: a Mendelian randomization study. Eur. Spine J. (2019). DOI:10.1007/s00586-019-06224-6
- Shadrina, A. S., Sharapov, S. Z., Shashkova, T. I. & Tsepilov, Y. A. Varicose veins of lower extremities: Insights from the first large-scale genetic study. PLoS Genet. (2019). DOI:10.1371/journal.pgen.1008110
- Zenin, A. et al. Identification of 12 genetic loci associated with human healthspan. Commun. Biol. 2, 41 (2019). DOI:10.1038/s42003-019-0290-0
- Sharapov, S. Z. et al. Defining the genetic control of human blood plasma N-glycome using genome-wide association study. Hum. Mol. Genet. 28, 2062–2077 (2019). DOI:10.1093/hmg/ddz054